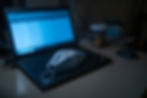
In my first article, we set some groundwork for interpreting information regarding Covid outcomes. In Part II, we looked at the effect of medical conditions on Covid outcomes. Since the dataset we used for examining the effect of medical conditions also included data regarding sex, race, and age, we can also examine the impact of those characteristics.
We’ve been using an analytical model called a Generalized Linear Model, which allows us to examine yes/no outcomes. In this case, we’re examining whether someone dies or does not die if they test positive for Covid. We are only including positive tests, and we are ignoring cases that are missing information.
In the last article, the variable examined had two values: medical condition or no medical condition. Since we were only dealing with two possible values, the explanation was simpler. This article will add a level of complexity by looking at characteristics that have multiple values. To do that, the model will need to make certain adjustments.
Computers and mathematical models cannot read. Everything they do is math. To make matters even more difficult, your computer can only do math with two digits, a zero and a one. Even something like Google Translate still needs to convert words to some numerical representation and back again. Our dataset has a column for sex with the possible values of male or female. We cannot do math on the words ‘male’ and ‘female.’ We have to do something to convert these words to numbers and then interpret those numbers in the context of their original meaning. These types of variables are referred to as categorical variables, since each value represents membership in a category.
There are a number of ways to convert categories to values. We’ll stick with the simplest for our model. Essentially, the model takes the column and turns it sideways, creating a new column for each category. For sex, it creates a new column for male and a new column for female. The model then uses zero in the column to represent ‘not’ in the category and one to represent membership in the category. So, if my record were in the dataset, I would have a zero in the female column and a one in the male column.
The second thing that the model must do is treat one column as the reference column. Our results then reference that column. Sex is a good column to start with, since it only has two categories. We will use female as the reference column, so the results for male are stated in relationship to outcomes for females. When we run the model, it gives us a value called the coefficient. A positive coefficient means greater likelihood, a negative means less likelihood. Since female is the reference, it is referred to as the intercept. The intercept in this case is negative. That tells us that females that test positive are less likely to die of Covid than the general population of people who test positive. The coefficient for males is positive, which tells us that males that test positive are more likely to die of Covid than females who test positive. Remember, female is the reference point.

In the first article, we used the dataset to calculate general odds for dying of Covid and then multiplied the result by a thousand, so we could deal in whole numbers. The general odds came out to 159 to 1000. For every 159 people that died of Covid, 1000 people survived. Looking at the influence of sex on Covid survivability, the model gives us odds of 198 to 1000 for men who have tested positive and 125 to 1000 for women who have tested positive. As expected, women are less likely to die from Covid, and men are more likely. The probability for men that have tested positive is 16.54%, and the probability for women is 11.13%. In this table, the odds ratio is male odds divided by female odds. You can think of them like percentages. Men that test positive have 58% higher odds to die from Covid than women who test positive.
As a reminder, these probabilities only apply to this dataset. We removed quite a few records that were unknown or missing data, so you can’t apply these to a general population. We are just looking to understand the relationships between characteristics and outcomes. In terms of scale, men are 50–60% more likely to die from Covid than women. Since the z-value is not between -3 and 3, and the p-value is less than 0.05, both categories measured are statistically significant.
This model doesn’t tell us anything about why, and it’s wrong to speculate. We could ask if men have more medical conditions. We could ask if the virus affects men’s physiology. Those would be areas of additional study. Later, we’ll look at the relationship between sex and existing medical conditions to see if they influence each other. At the moment, however, we don’t know, and we shouldn’t guess.
Besides a column for sex, we have a column for race. Race is also a categorical variable, but it has more values. The process remains the same. We end up with a column for each category under race with one column as the reference. We’ll use ‘White’ as the reference column. The other categories will provide likelihood in relationship to the ‘White’ category.

A couple of things to point out right away. The p-value for AIAN (American Indian/Alaskan Native) is greater than 0.05, and the z-value is between -3 and 3, so the AIAN characteristic is not statistically significant. Also, the coefficients for Hispanic and Islander are negative, which indicates that they are less likely than Whites to die of Covid. All of these statements come with some caveats. The count of AIAN and Islander categories is very small, so their inclusion in the model is questionable. It’s possible that a larger sample size could drastically change the results. In a real study, we would run it again without those categories and examine the impact. The Hispanic outcome is surprising. I know from other studies that many states do not report Hispanic cases. That could influence the results. Hispanic studies localized by state, county, or city might produce different outcomes. For our dataset, however, the Hispanic characteristic is less likely to die from Covid than White, and the category is statistically significant. We have to accept the findings for this dataset: Hispanics that test positive are about 35% less likely to die from Covid than Whites.
The rest of the table tells us that Asians and African Americans that test positive are about 18% more likely to die of Covid than Whites. Both values are statistically significant. We don’t know why, and we can’t speculate. Maybe it’s access to medical care. Maybe it’s physiological. Maybe it’s related to pre-existing medical conditions. We don’t know, and we can’t say from this data. We could look for answers by testing these possibilities with other datasets, but we cannot make any of those statements from this data.
What did we learn? We learned how categories work and what it means when you see a reference group in an article or graphic; we learned that men that test positive are more likely to die of Covid than women that test positive; and we learned that African Americans and Asians that test positive have a higher likelihood of dying from Covid than Whites do. Hispanics — surprisingly — showed a lower likelihood, which is an area that deserves further study.
In the next article, we’ll look at how age impacts Covid outcomes. Then we’ll wrap up by pulling all these variables together, seeing how they interact, and examining how the odds change as a result of those interactions.